Extended Data Fig. 3: The effect of key RNN parameters on performance.
From: High-performance brain-to-text communication via handwriting
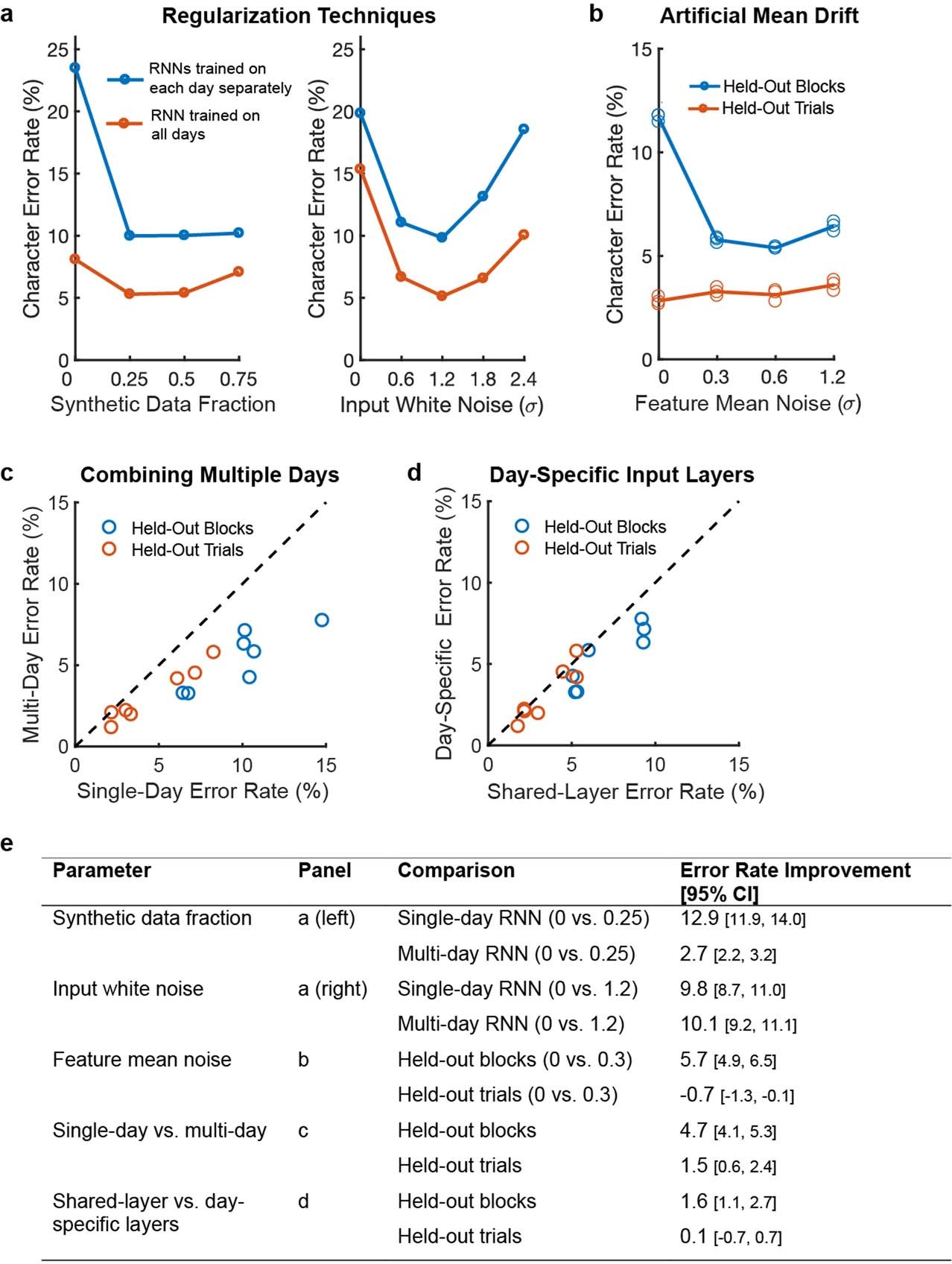
a, Training with synthetic data (left) and artificial white noise added to the inputs (right) were both essential for high performance. Data are shown from a grid search over both parameters, and lines show performance at the best value for the other parameter. Results indicate that both parameters are needed for high performance, even when the other is at the best value. Using synthetic data is more important when the size of the dataset is highly limited, as is the case when training on only a single day of data (blue line). Note that the inputs given to the RNN were z-scored, so the input white noise is in units of standard deviations of the input features. b, Artificial noise added to the feature means (random offsets and slow changes in the baseline firing rate) greatly improves the ability of the RNN to generalize to new blocks of data that occur later in the session, but does not help the RNN to generalize to new trials within blocks of data on which it was already trained. This is because feature means change slowly over time. For each parameter setting, three separate RNNs were trained (circles); results show low variability across RNN training runs. c, Training an RNN with all of the datasets combined improves performance relative to training on each day separately. Each circle shows the performance on one of seven days. d, Using separate input layers for each day is better than using a single layer across all days. e, Improvements in character error rates are summarized for each parameter. 95% CIs were computed with bootstrap resampling of single trials (n = 10,000). As shown in the table, all parameters show a statistically significant improvement for at least one condition (CIs do not intersect zero).